Goodgame Studios:
A modl.ai case study
Big Farm: Home and Garden is a free to play home design themed, match-3 puzzle game developed by Hamburg-based Goodgame Studios. They approached the team at modl.ai to see if there was a better solution using data-driven artificial intelligence (AI) to replace hours of development work.
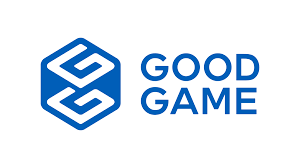
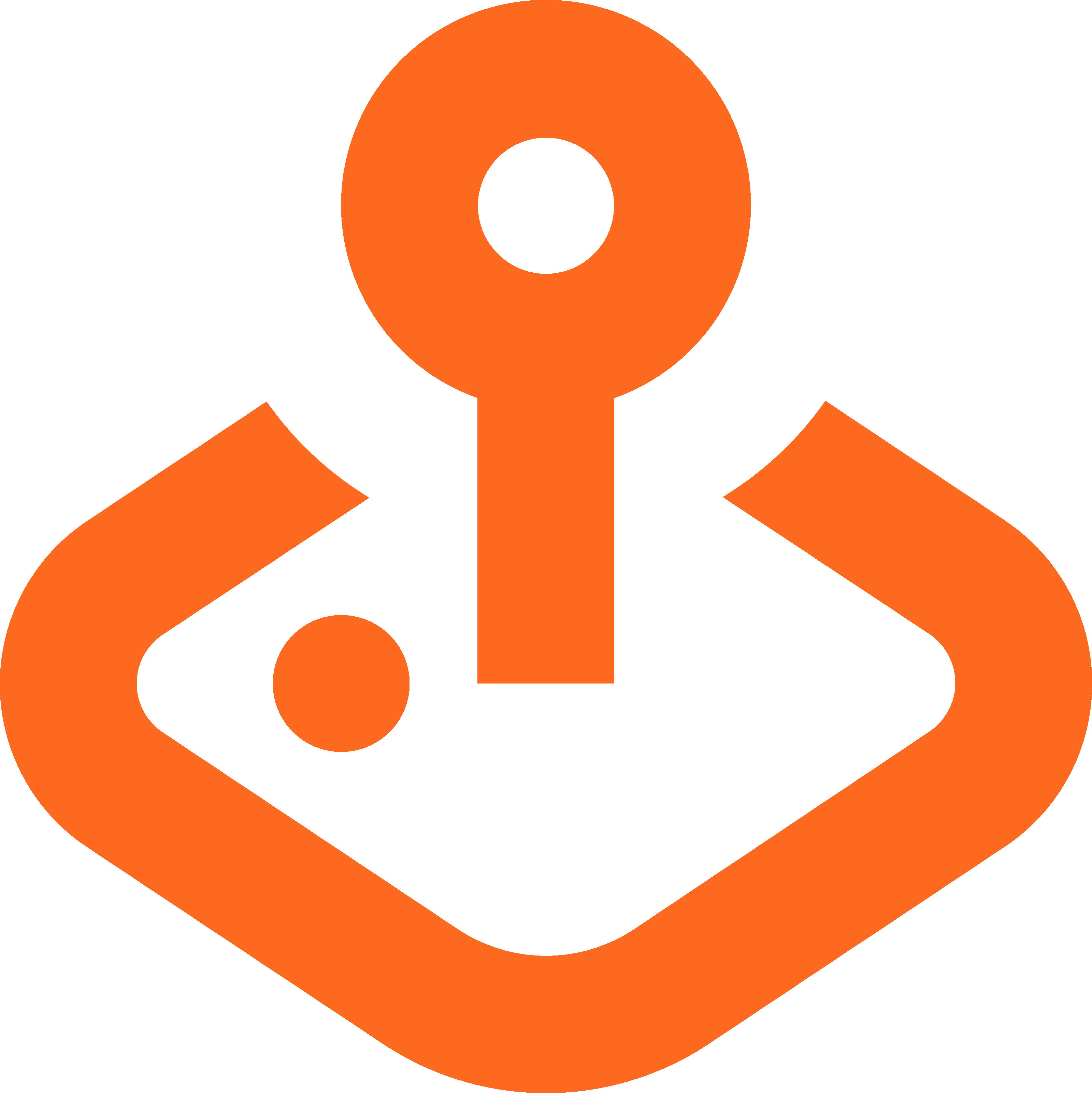
Platforms
Android
iOS

Game
Big Farm: Home and Garden
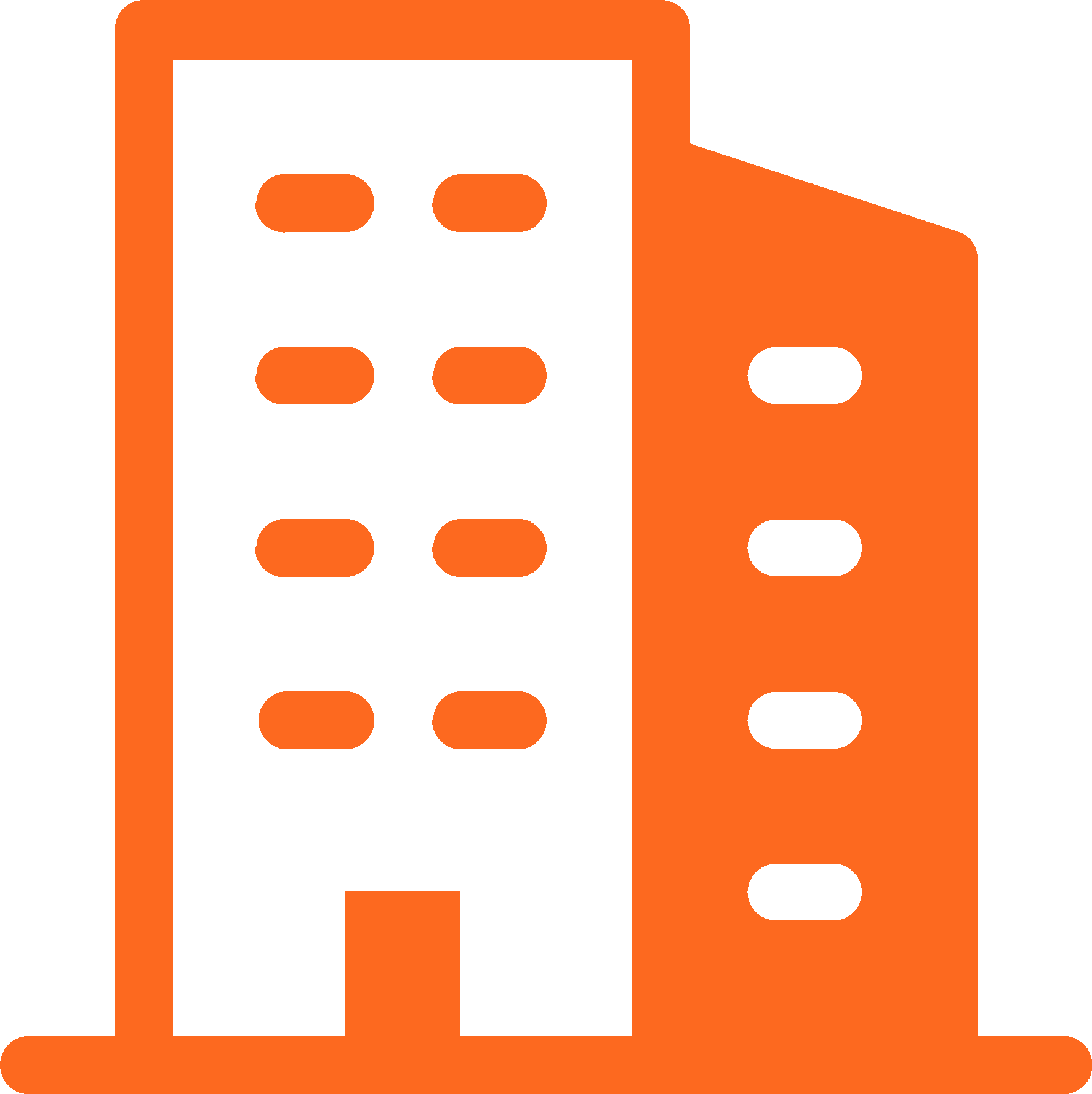
Company
Goodgame Studios is a leading developer and publisher of free-to-play games for web and mobile. Goodgame Studios was founded in 2009 by Kai Wawrzinek, Christian Wawrzinek and Fabian Ritter and has grown to become one of the biggest independent games companies in Germany.
Challenge
Match-3 games are known for having hundreds of levels to play, offering any level of player hours of entertainment. The obvious approach was to dedicate more developers to level design, but the team at Goodgame wanted a more scalable and less time-intensive solution.
Solution
We used modl AI Engine along with puzzle generator to make the level development process much faster. The team was able to launch the game on time with 16x more levels than what they would have been able to produce manually.
modl:create helped the team develop and release over 500 levels a week
“Creating new levels is really fast, we could have hundreds of new levels created overnight or during the weekends. Then come back the next day or next week, and just review them and select the best ones. With every new update, we can have many new levels without too much effort on our side or having to hire many level designers for the task.”
Guillermo Ruiz Campos, Project Manager
Goodgame Studio Brief
Big Farm: Home and Garden is based around a simple idea; players must solve a series of Match-3 puzzle games to transform crumbling farms or businesses with a design makeover. By solving puzzles, players can help design, customize and decorate their own farms with furniture, decor, and animals.
Interactive levels like those found in match-3 puzzle games are far more complex than they might appear, meaning that creating new levels is a highly skilled development task. Companies with successful puzzle games in their portfolio will typically have one or more dedicated level designers on staff. Even then, creating enough levels to keep players happy is an uphill task.
For Big Farm: Home and Garden to continuously provide players with new content, GoodGames Studios realized it would need to hire more level designers or look for a way to speed up the level design process. That’s when they reached out to modl.ai.
Our Approach
Puzzle games are often based on levels with one specific solution, but with match-3 games, there can be many ways to complete a level successfully. An innovative way of using AI for data-driven level generation or learning-based level generation is to train the model with pre-existing levels until it understands the relationships between the different design elements. Using this data, the AI essentially learns the patterns present in each level, which can then be reproduced in slightly different ways.
We looked at procedural content generation via machine learning (PCGML) – a method that allows the AI to learn from existing content and produce similar content automatically. We quickly found that while PCGML worked well with static images, however, it was less effective with interactive match-3 levels when used on its own. The challenges lie in replicating player behavior, functionality constraints, interactive mechanics, and aesthetics.
These challenges led us to change our approach. We decided to create an AI-based system consisting of two distinct parts: a machine learning-based level generator and a level evaluator using modl AI Engine.
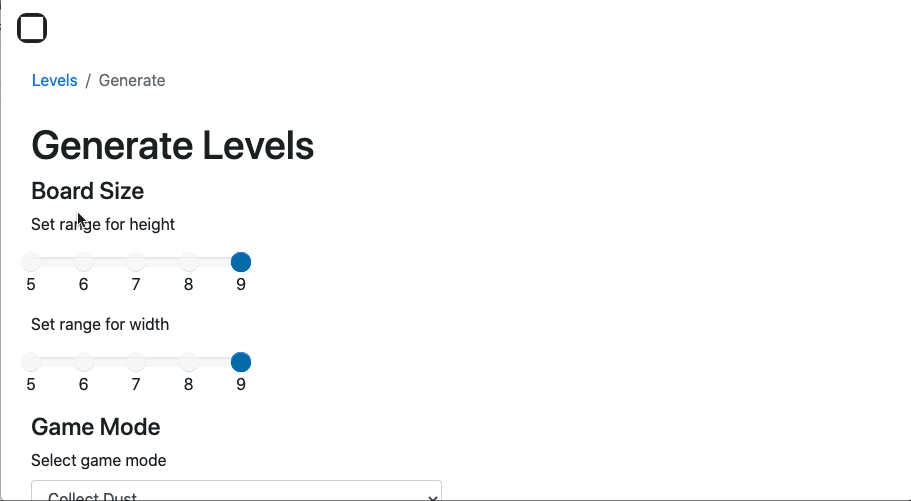
We found that whilst AI allowed us to create lots of varied levels, there was no easy substitute (yet!) for the experience and expertise of human-level designers in knowing what makes a level fun to play.
So we decided to stay away from purely data-driven methods and give a degree of control to the level designers, so they would have the ultimate decision on whether a level would make it into the final game or not by considering different aspects such as bot winrates and winrate predictions by the AI.
Final Result
Our solution was the result of many weeks of work on this interesting challenge. The level generator gives level designers complete control over selected aspects of the design through a dashboard where different design parameters can be entered, including the dimensions, goal parameters, and type of pieces to include in each level.
modl AI Engine is dedicated to testing the generated levels. Multiple bots trained by using pre-existing match-3 levels, play each new level and characterize it based on features such as how many moves it takes to complete, the time it takes to complete and the number of reshuffles required. All of these outputs give the designer an insight into the difficulty and structural features of the level. Developers can also upload their handcrafted levels, which the bot will evaluate using the same system.
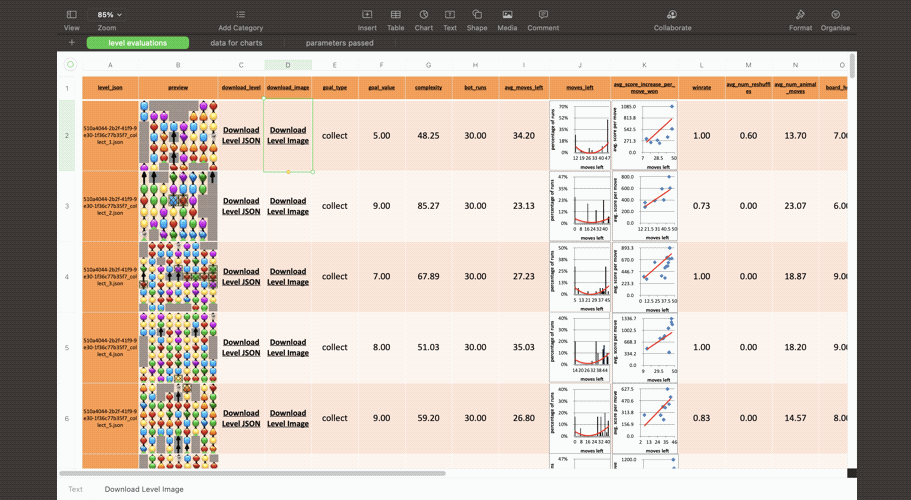
Peer Kröger, a Game Designer for Goodgames Studios, is thrilled with how the tool has helped the team save huge amounts of time and effort while giving fans of the game lots of new content to keep them engaged.
“The generation is fast, flexible and convenient to use. It allows us to add a much higher amount of puzzles to the game compared to crafting them by hand. Overall, we are increasing the amount and the frequency of the content that we can release for our game.”
Peer Kröger, Game Designer
Our tool will output a level as code ready to be added if the level designer thinks a level is good enough to be added to the game. The result is a platform that can easily generate 50 levels in a day, tested and ready to be deployed, allowing Goodgames Studios to launch the game sooner and with a smaller team.