We met with veteran game executive Ben Wibberley to discuss how AI can empower QA teams and build better games.
As a three-time founder with nearly three decades of experience in QA, global production, and live quality operations, Ben Wibberley is a games industry veteran. He’s served as the VP of Games Operations at Jagex and founded Digital Age Quality Assurance in 2017, for which he now acts as Managing Partner. We were lucky enough to sit down with Ben to discuss how he sees AI enhancing the games QA process and ultimately improving the player experience.
His approach highlights three essential pillars in modern QA: preserving the “last known good,” efficiently managing new content, and using player insights to focus QA on the areas that matter most.
Key Takeaways
- Preserve stability with “Last Known Good”: Use AI-driven automation to validate that updates don’t disrupt core game elements, maintaining the stability of previous builds as new features are rolled out.
- Augment QA for rapid content releases: AI can handle repetitive, high-volume testing tasks, allowing human QA teams to focus on more complex issues and keep up with modern games’ fast-paced release cycles.
- Leverage player insights to enhance QA focus: By analyzing player feedback through AI, studios can prioritize fixes for player experience issues, making QA efforts more player-centered and retention-driven.
1. Maintaining the last known good: AI as a safety net
Ben starts by introducing the concept of reserving the “last known good” (LKG) version — a concept taken from the world of operating system configurations — that represents a stable baseline of the game. He explains how it is critical as new features and content are introduced. For live-service games in particular, AI-driven automation tools ensure that updates don’t destabilize this core version. By continuously validating the LKG state, automation enables QA to act as a “safety net” for each update, catching issues early before they reach production.
“With automation — whether at the code level or content level — you can release new content with confidence, knowing it won’t disrupt your last known good. Automation provides that essential layer of assurance, continuously verifying that the core game experience remains intact as updates roll out. This lets QA teams catch issues early, protecting stability and allowing us to focus on delivering quality enhancements rather than revisiting past fixes.”
In the most sophisticated games, automation’s role in LKG maintenance goes beyond stability; it allows studios to identify issues earlier in development, reducing costly late-stage fixes. Automated testing continuously validates key mechanics, preventing cascading issues from minor errors that might otherwise go unnoticed.
Ben emphasized that automating these checks eases the burden on QA teams, allowing them to focus on polishing new content rather than revalidating existing features. This LKG-focused approach enables studios to iterate faster while reducing risks, supporting a smoother, more reliable development cycle.
2. Keeping up with new content velocity: AI-augmented QA for rapid iteration
Modern games operate at a pace unimaginable even a few years ago, with new content, features, and updates dropping frequently. Modern games like Fortnite release updates at a blistering pace as they embrace the games-as-a-service (GaaS) model. This continuous influx of new content puts immense pressure on QA teams to ensure thorough testing on tight timelines. Ben sees a solution in AI-driven tools that augment human testers, allowing teams to keep pace with production without sacrificing quality.
“The speed and volume of content releases now are staggering. AI’s value is in augmenting our teams so they can work smarter and faster, validating decisions and handling the rapid pace of new content. By handling repetitive tasks and scaling up our testing efforts, AI lets us focus on complex, experience-driven testing that elevates gameplay without being held back by the volume of updates. It’s about enabling QA to keep up without compromising quality.”
AI’s capability to run large-scale simulations and stress tests, such as load testing for multiplayer servers or unique scenario testing, enables QA teams to efficiently manage the demands of today’s production pipelines. “AI-based tools free up testers to tackle what machines can’t,” Ben added, emphasizing that this division of labor allows QA pros to address nuanced game mechanics while AI handles the more repetitive workload.
3. Player insights: Turning feedback into actionable QA data
Listening to player insights is essential for a truly player-centric QA process. By using AI to analyze feedback from reviews, in-game behavior, and community forums, QA teams can pinpoint and prioritize the issues that players care about most. This feedback loop is crucial for long-term retention, helping teams quickly address bugs, exploits, and frustrating gameplay elements that might otherwise go unnoticed.
“Retention today is driven by understanding what players value — and just as crucially, what they dislike. They hate bugs, cheating, and connectivity issues, and if we address these quickly, we’re more likely to keep them engaged. AI can help surface these frustrations from player feedback, forums, and reviews at scale, allowing us to prioritize fixes that directly impact player experience. When we focus QA efforts on what matters most to players, we’re not only fixing issues; we’re building trust and ensuring a smoother, more enjoyable experience.”
AI tools that can aggregate and interpret vast amounts of player feedback allow studios to pinpoint problem areas more efficiently and respond directly to player needs. Ben explained that automated systems can sort through thousands of reviews and forums — even across different languages — surfacing common pain points and providing actionable insights for QA teams.
“When AI can surface common pain points directly from players, QA teams can respond proactively, which enhances player trust and keeps them engaged,” Ben explains. This data-driven, player-centric approach prevents technical issues and enhances the player experience, creating a QA process that prioritizes quality and player satisfaction while bolstering retention.
AI as a strategic partner in modern QA
Ben’s insights highlight the transformative potential of AI as a strategic QA tool. For studios looking to integrate AI effectively, Ben underscores the need for a balanced, player-centred approach that leverages AI’s strengths without replacing human intuition.
“AI won’t replace our QA teams; it will enhance them. AI is crucial for where we’re heading, but it’s not a standalone solution. You have to see it as part of the bigger picture.”
As the industry continues to evolve, studios that thoughtfully embrace AI in these key areas will be better equipped to deliver high-quality, engaging experiences to players. For studios ready to put these principles into practice, exploring AI-driven testing tools like those from modl.ai is a great place to start in building a more resilient, player-focused QA pipeline.
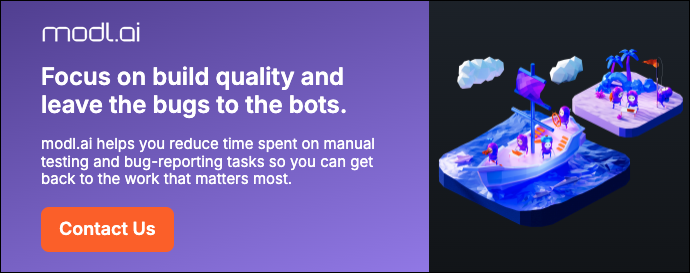